Abstract
We are happy to present this joint work with Mihaela Roșca, Răzvan Pascanu, Lucian Bușunoiu and Claudia Clopath on the effect of spectral normalisation in deep reinforcement learning.
Most of the recent deep reinforcement learning advances take an RL-centric perspective and focus on refinements of the training objective. We diverge from this view and show we can recover the performance of these developments not by changing the objective, but by regularising the value-function estimator. Constraining the Lipschitz constant of a single layer using spectral normalisation is sufficient to elevate the performance of a Categorical-DQN agent to that of a more elaborated Rainbow agent on the challenging Atari domain. We conduct ablation studies to disentangle the various effects normalisation has on the learning dynamics and show that it is sufficient to modulate the parameter updates to recover most of the performance of spectral normalisation. These findings hint towards the need to also focus on the neural component and its learning dynamics to tackle the peculiarities of Deep Reinforcement Learning.Bio
Florin Gogianu is a member of Bitdefender's Machine Learning & Cryptography unit and a PhD student at the Technical University of Cluj-Napoca, Romania. His main interests revolve around the problem of learning good representations for Deep Reinforcement Learning, leading to agents with better generalization properties and improved sample complexity.
Tudor Berariu is a PhD student at Imperial College London, researching plasticity of neural networks. He is a member of the Clopath lab, being supervised by Claudia Clopath and Răzvan Pașcanu. His main research interests are in the areas of continual learning and optimisation of deep neural networks.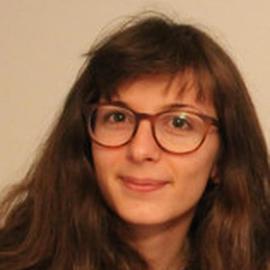