Abstract
Probabilistic modeling is a core component of modern machine learning. Nevertheless, at least from our local perspective, educational barriers and practical implementation issues have undermined its applicability in both academy and industry. In practice, this vacuum is often filled by black-box approaches such as traditional neural networks. In such a scenario, our group (LOGIA) aims to provide some basis to enhance teaching, research and applications of probabilistic machine learning methods in our local community. In this talk we present some of our current work on the area, ranging from the proposition of new models to extensions and applications of consolidated approaches. We hope that our investigations will help to overcome limited local adoption of those methods, as well as contribute to the global ML community.Bio
César Lincoln Cavalcante Mattos has been an associate professor at the Department of Computer Science, at Federal University of Ceará (UFC), Brazil, since 2018. He is also an associate researcher at the Logics and Artificial Intelligence Group (LOGIA). He has research interests in the broad fields of machine learning and probabilistic modeling, such as Gaussian processes, deep (probabilistic) learning, approximate inference and system identification. He has been applying learning methods in several research and development collaborations in areas such as dynamical system modeling, health risk analysis, software repository mining and anomaly detection.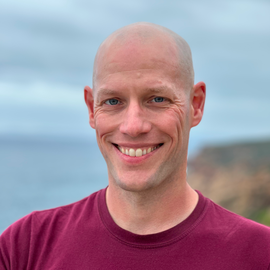
Google DeepMind Chair of Machine Learning and Artificial Intelligence