Bio
Ti is a senior machine learning researcher in the probabilistic modelling team at Secondmind Labs, where they have been working on a broad range of customer and research projects involving Gaussian processes. Ti believes in making research output reusable by integrating it in common toolboxes and is core maintainer of the GPflow open source project for Gaussian process modelling.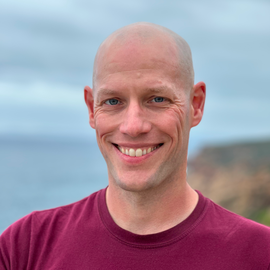
Google DeepMind Chair of Machine Learning and Artificial Intelligence