Bio
Rituraj Kaushik is a 4th year Ph.D. candidate on Machine Learning & Robotics at INRIA-Nancy (Université de Lorraine), France. His research activity is focused on designing model-based robot learning algorithms to allow a robot to adapt to unanticipated situations such as damages within a few minutes of interaction using prior knowledge derived from simulators. He received his master's degree in Electronics Design & Technology in 2016 from Tezpur Univerity, India.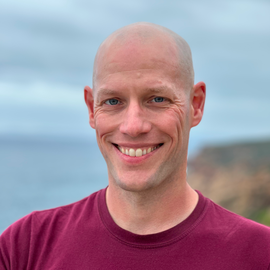
Google DeepMind Chair of Machine Learning and Artificial Intelligence