Bio
Vincent Adam studied engineering and cognitive science in Paris before completing a PhD at the Gatsby Unit, UCL in between machine learning and computational neuroscience. Initially using the framework of Probabilistic modelling as a normative model for cognitive function (perception), he currently uses the same framework to develop interpretable and scalable machine learning algorithms at Prowler.io, with a focus on Gaussian Processes and time series modelling.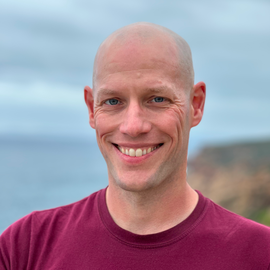
Google DeepMind Chair of Machine Learning and Artificial Intelligence