Bio
Currently, I am a PhD student at KTH (at the Robotics, Perception & Learning division in the group headed by Danica Kragic). My research is focused on constructing simulation-based kernels to improve data efficiency of Bayesian optimization (BO) for dynamic
manipulation tasks, task-oriented grasping and locomotion. More generally, I am interested in reinforcement learning for robotics domains with a challenging sim-to-real gap. Previously, I was a research Masters student at the Robotics Institute at Carnegie
Mellon University. At CMU I worked on BO for bipedal locomotion (with Akshara Rai and Chris Atkeson) and on improving data efficiency of BO for personalized education systems (with Emma Brunskill - my MS adviser). Earlier, I was a software engineer at Google.
I started in the Personalized Search team in core ranking, then joined the Character Recognition (OCR) team and worked on the open-source OCR engine Tesseract, which was used for Google Books and StreetView.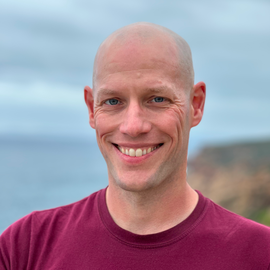
Google DeepMind Chair of Machine Learning and Artificial Intelligence